Zihao Zhu
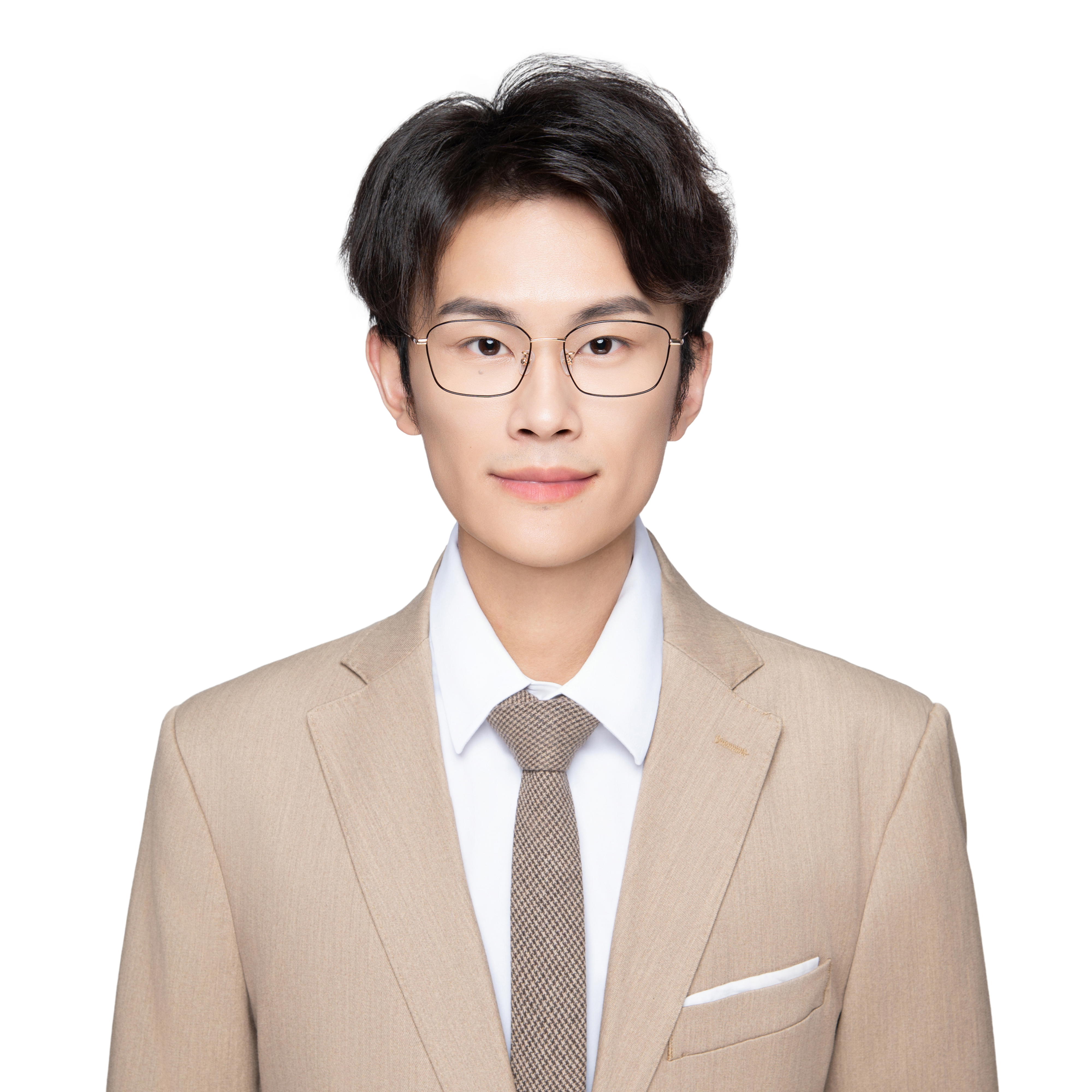
zihaozhu@link.cuhk.edu.cn
Hi, this is Zihao Zhu (朱梓豪). I am currently a Ph.D. student in Data Science at The Chinese University of Hong Kong, Shenzhen, under the supervision of Prof. Baoyuan Wu. Previously, I received my Master’s degree from the Institute of Information Engineering at the University of Chinese Academy of Sciences in 2021.
My research interests lie in the broad area of AI security, with focuses on three main directions:
- Security of Large Language Models: I work on understanding and addressing security challenges in LLMs, including jailbreak attacks and AI alignment. This research aims to make language models more robust while maintaining their utility.
- Data Security in AI Systems: Data is the fuel of AI. I investigate various aspects of data security in Data-centric AI (DCAI), with particular emphasis on backdoor attacks and data quality assessment.
- Security in Embodied AI: I explore security concerns in embodied AI systems, focusing on risk assessment for AI agents. This emerging area is crucial as AI systems become more integrated into physical environments.
If you share similar interests, please feel free to reach out. I am happy to chat and open to exploring opportunities for collaboration.
I am currently on the job market and seeking full-time opportunities in academia or industry. I would be delighted to connect if you have relevant openings or suggestions.
news
Mar 29, 2025 | Our paper “BackdoorBench: A Comprehensive Benchmark and Analysis of Backdoor Learning” has been accepted to IJCV! ![]() |
---|---|
Feb 19, 2025 | Our new paper “BoT: Breaking Long Thought Processes of o1-like Large Language Models through Backdoor Attack” is available on arXiv. Check out the code on GitHub. |
Dec 07, 2024 | One new preprint is available: “HMGIE: Hierarchical and Multi-Grained Inconsistency Evaluation for Vision-Language Data Cleansing” ![]() |
Jan 20, 2024 | Our paper “Learning to Optimize Permutation Flow Shop Scheduling via Graph-based Imitation Learning” has been accepted to AAAI 2024! ![]() |
Jan 20, 2024 | Our paper “VDC: Versatile Data Cleanser based on Visual-Linguistic Inconsistency by Multimodal Large Language Models” has been accepted to ICLR 2024! ![]() |